A “bottom up” and integrated data quality approach
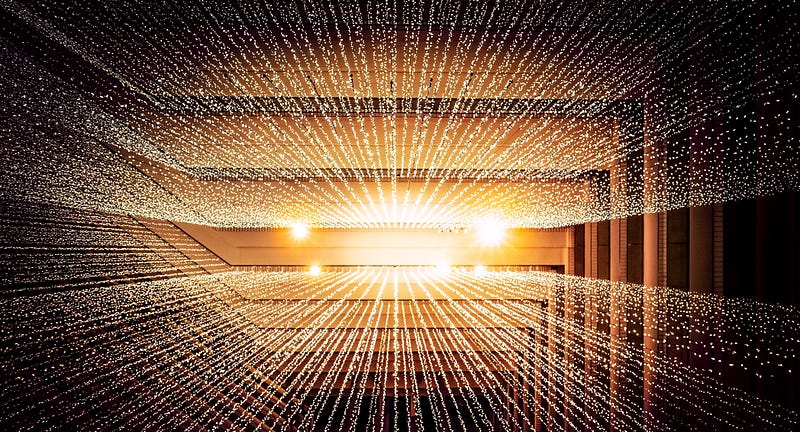
For a data treatment plant
Whilst acknowledging that the financial industry has become conscious of its “data urgency”, current operational processes and staff are still struggling from a mass of dirty data that not only impacts the business accuracy, but also drowns their value at work and undermines their motivation.
As a recent HBR study shows, “47% of newly created data records on average have at least one critical work-impacting error” which can lead up to “10 times more expense to compete a unit-of-work”.
Why are we always willing to build a house from the roof?
To build their data strategy, most organizations have started by reorganizing and often centralizing their data operations and information technology. They have created new functions such as data stewards and chief data officers (CDOs), implemented top down data governances and data management empirical methodologies.
If 85% of respondents to a NewVantage Partners survey say they started their data-driven journey, 37% only report success so far pointing management understanding, organizational alignment, and general organizational resistance as the culprits.
STOP!
You should overstep the traditional “lead from the top” approach. Instead, adopt a “bottom up” approach: it should start from a specific operational process and create a cleaning loop where data is cleansed based on Machine Learning capabilities empowered with human feedbacks.
Everything starts with people
There is a need to first develop staff skills and knowledge around data.
This awareness is crucial as it will enable them to understand data lifecycle and state of transition. They will be able to grasp the ins and outs of complex data environments; the cost of poor data, the money wasted on unmanaged data and the loss of business opportunities.
Start from the basis…
Now that you have trained “data people” ready to work, you need to identify quick wins that will support your data transformation and be a real potential of improvement.
Indeed, a strong data foundation begins with the understanding of data, their decay, their quality dimensions (aspects we want to measure), and how to prioritize improvement efforts and make a business case for it.
To do so, you should definitely use what technology has to offer: get the facts you need with minimum effort.
Data discovery helps to quickly and easily discover outliers and detect patterns in operational processes. Hence the data that need an urgent treatment plant.
The objective is to firstly clean up these existing processes, to free people from their noisy data burdens, and empower them so they can better monitor and analyze their current business, take the right decisions, have innovative ideas and of course embed this data culture.
… and create a virtuous cycle
The challenge is to feed your data quality rules with as many business knowledge as possible. The point is to have them operate efficiently so that operational teams’ time is spent on the exception of the exception.
Use Machine Learning algorithms and enrich them with human feedbacks.
Data quality checks, augmented by users’ feedback loops, is central to the success of any operation and its value will increase over time.
The constant interaction between you and your data will help you optimize your data assets, work more efficiently and improve your processes towards an operational excellence.
Convert the try — build on trust
Data quality management is just one brick of a global data foundation and it has to be based on a robust methodology.
Other elements will also have to be considered such as the data architecture, data governance, data analytics strategy, data visualization and last but not least a data-driven culture.
After that, you will be in the right position to understand the value of the data you possess, pinpoint new potential revenue streams and try to turn them into reality.
References
Carl Anderson — Creating a Data-Driven Organization
Martijn Groot — A primer in Financial data management
Mike Fleckenstein / Lorraine Fellows — Modern Data Strategy
Pwc — Data & Analytics
HBR — Only 3% of Companies’ Data Meets Basic Quality Standard — September 11th 2017
NewVantage Partner — Big Data Executive Survey 2017
For more information : @BittnerPierre